Approach
The first goal was to help Proximus choose the best database for Scope 3 emission factors. To do this, Möbius made a clear comparison between different providers. Each one was checked based on important points like how often they update their data, how detailed the data is, and how they calculate emissions.
A critical aspect here was the availability of supplier-specific emission factors. While the majority of the providers only provide emission factors on industry category levels (such as NACE or GICS classifications), others also provide these specifically on the supplier level. A coverage check showed that many of Proximus’ suppliers were included in these detailed databases. This means using this more specific data could lead to more accurate results.
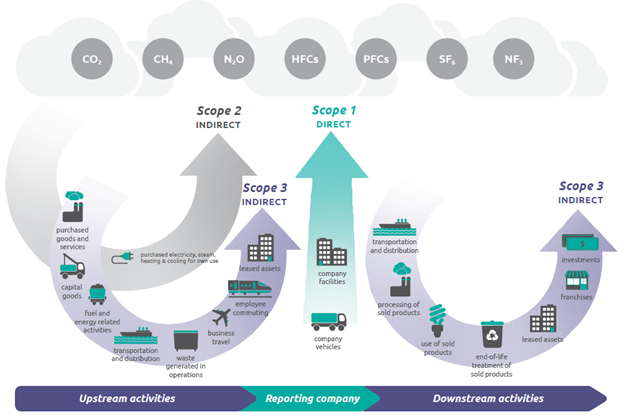
After the new data provider was chosen, a structured and sustainable codebase was developed in Databricks, which allowed for easy access to the necessary databases with the emission factors of the providers. The approach of Möbius consisted of 4 steps:
1. Supplier identification mechanism
The supplier data from Proximus' procurement team didn’t always match the names or codes used in the emission database. So, Möbius created an automated tool to connect the dots. It matched suppliers using VAT numbers or AI-based matching (looking at name, country, and other details). We also built a check to review and adjust these matches, especially for the biggest suppliers.
2. Supplier-level Emission Factor gathering
Once we had the suppliers matched, the next step was to pull in the actual emission factors. In Databricks, we wrote code to gather this data both at the 'Child' (the local entity) and the 'Parent' (holding) level. Since emissions can differ between a local branch and its parent company, it was important to have both.
3. Industry-level Emission Factor gathering
Some smaller suppliers don’t report their emissions. For them, we needed another way. So, we used their NACE codes (a European industry classification) to figure out what kind of business they’re in. Then, we translated that into GICS codes (another industry standard) to get average emissions data for that industry. This gave us a reasonable estimate for suppliers without specific data.
4. Decision making & logic
We set up rules so the system always picks the most detailed and accurate data available—whether that’s from the parent company, the local supplier, or the industry average. This means each supplier’s emissions are calculated using the best possible information.
One challenge is timing: companies often report last year’s emissions in this year’s reports, and industry averages may lag even further behind. To deal with this, Möbius has built a mechanism that made the necessary corrections for inflations, based on the latest available information for both company & industry level Emission Factors.
Results
To make things as user-friendly as possible, all final calculations and emissions reports were delivered in a well-structured Excel file. This file wasn’t just for viewing results, it allowed Proximus to make manual adjustments to the decision-making logic if needed. Any changes made here had an immediate effect on the calculations, giving full control and transparency over the results.
The Excel file gave a complete breakdown of CO₂ emissions per supplier, using the most accurate emission factors available—whether based on specific supplier data or industry averages. Thanks to this detailed output, Proximus now has a much clearer view of how its spending links to carbon emissions, and how each supplier contributes to their total footprint.
This wasn’t just a one-time exercise. With the Möbius approach, the entire process can be easily repeated year after year. This calculation not only helps Proximus for setting the baseline & SBTi-based targets, but also for keeping track of the future performance.
Möbius played a crucial role in making this happen, guiding Proximus through the technical complexity and building a flexible solution that will support them for years to come.